- Tune AI
- Posts
- š§š Unlike Rest, Meta Making Their Own Data
š§š Unlike Rest, Meta Making Their Own Data
ā²ļø OpenAI Partners for New Data, AMD Gears up for the Long Game, and AWS Fixes your RAGs

Hello Tuners,
In the latest tech twists and turns, the AI landscape is buzzing with game-changing developments. AMDās acquisition of ZT Systems is set to supercharge its AI infrastructure with a strategic play to blend top-notch design and deployment prowess. Meanwhile, Amazonās RAGChecker is revolutionizing how we evaluate AI systems, ensuring they sift through the data deluge with surgical precision.
Not to be outdone, the Phi-3.5-mini is proving that more minor can be mightier in the AI arena, showcasing impressive multilingual and reasoning chops despite its compact size. In a nod to innovation, a new RAG system model optimises multi-hop question-answering by slashing redundant LLM calls and curating knowledge with a deft touch. The AI realm is evolving, and witnessing these transformative strides unfold is thrilling!
Sam Altman-led OpenAI has announced a multi-year partnership with CondƩ Nast, bringing content from renowned brands like Vogue and The New Yorker into its AI products, including ChatGPT and the newly launched SearchGPT. This move follows similar agreements with other major media outlets such as Time, Financial Times, and Le Monde, reflecting a growing trend of integrating high-quality content into AI-driven platforms.

However, the partnership comes amidst ongoing controversies. OpenAI and other AI companies like Perplexity have faced accusations of scraping data from platforms without permission, leading to legal challenges from media organizations like The New York Times. As AI continues to play a larger role in content delivery, these partnerships highlight the delicate balance between innovation and the ethical use of data.
Patching With Houston, Deploying Tune Assistant! š§āš
Tune Assistant is now live in production, showcasing a range of advanced features designed to boost its functionality and integration capabilities. Hereās a rundown of the new features:
Define Custom Instructions: Tailor the assistantās tasks to your specific needs.
Custom Function Model: Select a model to determine the appropriate function to call.
Custom Response Model: Merge outputs from various tools into a unified response.
Web Search: Instantly fetch answers from the web.
Image Generation: Create stunning visuals on the fly.
Actions (OpenAPI spec): Connect and interact with external applications.
Function-Calling (10x smarter): Access real-time data and execute custom functions.
Head to Tune Studio Now to get the most out of your Models and Credits! š

In the world of Large Language Models (LLMs), human evaluation has always been the gold standardāuntil it started feeling more like gold-plating: costly, time-consuming, and requiring a level of expertise that not every team has on hand. But Meta FAIR seems to have had enough of waiting for humans to catch up. Enter the Self-Taught Evaluator, an AI that trains itself using synthetic data, bypassing human annotations. Itās like giving your AI a stack of books and telling it to become its teacher. Cool, right? Well, sort of. The method isnāt without its quirks, and while it promises to make LLM evaluation faster and cheaper, itās still a gamble whether this self-educated AI will pass the real-world test.

Meta FAIRās Self-Taught Evaluator starts with a seed model, feeds it with unlabeled human-written instructions, and lets it play judge, jury, and executioner. The AI picks the āchosenā and ārejectedā responses, training itself on what it thinks is right. And guess what? It worked well, boosting performance on benchmarks like RewardBench and MT-Bench without a single human lifting a finger. It sounds like a win for AI autonomy, but hereās the rub: this approach could optimize models for test scores rather than real-world tasks. So, while itās a tempting tool for enterprises looking to fine-tune models on massive unlabeled datasets, the truth is, thereās no skipping out on manual testing if you want your AI to do more than ace its homework.
AMD dropped $4.9 billion to bring ZT Systems into its AI arsenal, marking yet another step in its mission to dominate the AI infrastructure game. ZT Systems, known for its magic in designing AI infrastructure for hyperscale data centres, is now part of the AMD family. This deal isnāt just about adding a fancy new company to the roster; itās about turbocharging AMDās AI offerings. By blending AMDās Instinct AI accelerator and EPYC CPU with ZTās expertise in cloud computing solutions, AMD is poised to deliver AI infrastructure at a scale thatās as big as its ambitions. The idea? To make sure that when the worldās largest cloud companies need to scale up, they do it with AMD-powered systems humming in the background.

While AMD and ZT Systems are all set to become best buds, thereās more than meets the eye here. After the dust settles, AMD plans to offload ZTās manufacturing business to a strategic partner. Itās a classic ākeep the brain, sell the brawnā move. With ZTās CEO, Frank Zhang, leading the manufacturing side, and President Doug Huang heading design and customer enablement, AMD is keeping the talent it needs while shopping around for someone else to handle the nitty-gritty of manufacturing. By the end of 2025, AMD expects this deal to start paying off on a non-GAAP basis, making it clear theyāre not just playing for today but building a future where they control the full AI stack. So, while the acquisition may sound like another line in a press release, itās a strategic power play in the fast-moving world of AI infrastructure.

Amazon's AWS AI team has introduced RAGChecker, a cutting-edge tool to tackle a crucial challenge in AI: ensuring the accurate retrieval and integration of external knowledge into AI-generated responses. RAGChecker is designed to enhance Retrieval-Augmented Generation (RAG) systems, vital for AI applications requiring up-to-date and precise information, such as in medicine or finance. Unlike traditional evaluation methods, RAGChecker breaks down AI responses into individual claims, providing a granular analysis that helps identify specific errors in retrieval and generation phases, potentially making AI more reliable in high-stakes environments.

RAGChecker isn't just a research tool; it's also a powerful asset for enterprises looking to refine their AI systems. It offers overall metrics for comparing different RAG systems and diagnostic metrics pinpointing weaknesses. Amazon's testing of RAGChecker across multiple domains revealed trade-offs between retrieval accuracy and information relevance, highlighting areas for improvement in AI reasoning capabilities, especially in open-source models.

LLM Of The Week
Phi-3.5-Mini
Phi-3.5-mini, a compact variant of the Phi-3 model family, excels in multilingual capabilities and long-context understanding. Trained on a diverse dataset that includes high-quality educational material, code repositories, and synthetic data, the model has undergone rigorous fine-tuning to ensure precise instruction adherence and robust safety. Supporting a wide range of languages, Phi-3.5-mini performs impressively on multilingual benchmarks, holding its own against larger models like GPT-4, particularly in tasks requiring long-context processing, such as document summarization and information retrieval.
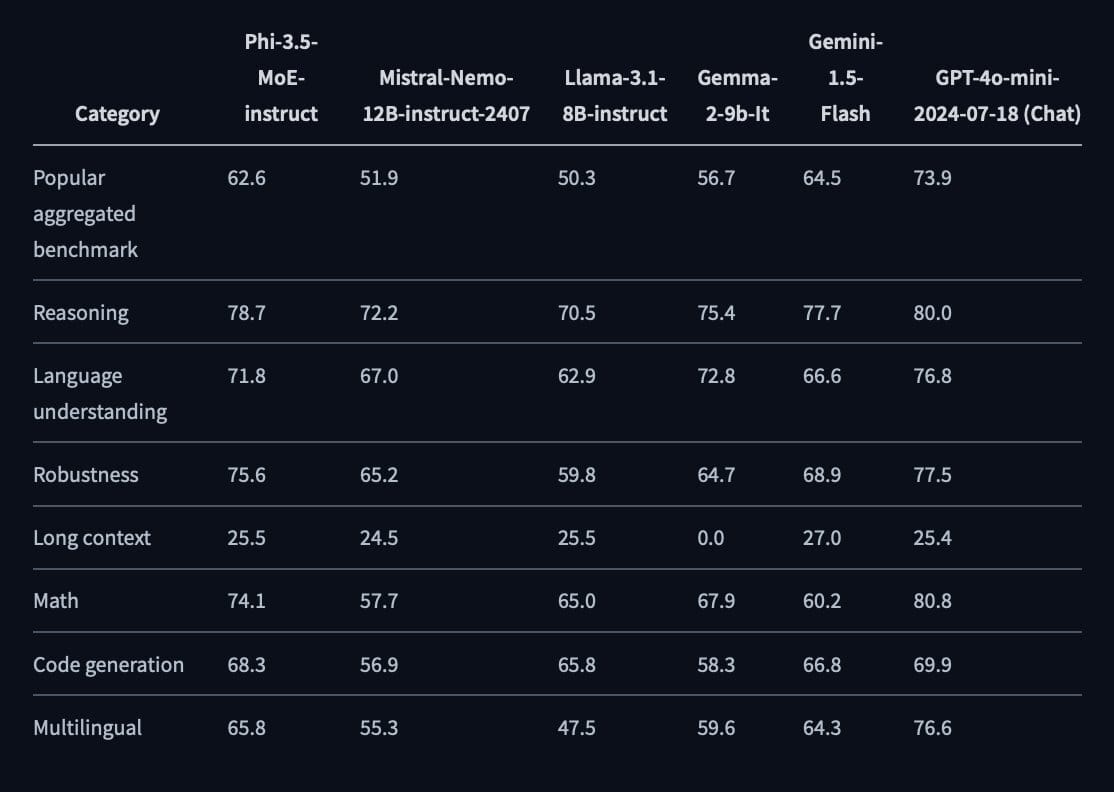
Despite its smaller size, Phi-3.5-mini demonstrates strong reasoning and problem-solving abilities across various domains, often coming close to the performance of much larger models. Its efficiency in handling extended text sequences and tackling complex tasks makes it a versatile tool, suitable for various applications in AI-driven language processing, even though it doesnāt match the top-tier performance of models like GPT-4 on some benchmarks.

Weekly Research Spotlight š
EfficientRAG: Efficient Retriever for Multi-Hop Question Answering
This innovative approach to improving the efficiency of Retrieval-Augmented Generation (RAG) systems revolves around two key components. The first component involves training an auto-encoder language model (LM) to label and tag chunks of retrieved information. By marking these chunks as either <Terminate> or <Continue> and annotating <Continue> chunks for further processing, the system ensures a streamlined retrieval process. The second component is a filter model, which iteratively formulates next-hop queries based on the original question and previous annotations. This iterative approach continues until all chunks are appropriately tagged or a maximum number of iterations is reached.

Combining these components creates a highly efficient system for multi-hop question-answering, significantly reducing the need for multiple LLM calls typically required in RAG systems. This method effectively curates relevant knowledge while minimising noise and irrelevant information by utilizing synthetic data from an LLM to train the labeller and filter models. This paradigm shift in RAG systems enhances accuracy and efficiency, particularly in complex, multi-hop QA tasks requiring precise and contextually relevant information.

Best Prompt of the Week šØ
A green box of skin care products stands on an island in the middle, surrounded by water and moss-covered stones. The sky is clear with a large white circle above it. A bird perches on top of one stone, adding to its natural beauty. Above all these elements hangs a large advertising poster. --ar 37:56 --s 250 --v 6.1

Today's Goal: Try new things š§Ŗ
Acting as a Skill Development Planner
Prompt: I want you to act as a skill development planner. You will create a structured daily plan designed to help individuals enhance their vocal abilities and rap skills. You will identify a target client profile, develop key strategies and action plans, select the tools and resources for effective vocal training and rap practice, and outline any additional activities needed to ensure consistent improvement. My first suggestion request is: "I need help creating a daily activity plan for someone who wants to improve their vocal skills and rap abilities.
This Weekās Must-Watch Gem š
This Week's Must Read Gem š
How did you find today's email? |