- Tune AI
- Posts
- ⚖️OpenAI Grabs 'o1' as Clone Wars Brew!
⚖️OpenAI Grabs 'o1' as Clone Wars Brew!
🔮Qwen's Model Rivals GPTo1, Google Creates Chess Assistant and MCP Connecting Cloud with Anthropic

Hello Tuners,
This week in GenAI, Alibaba's QwQ-32B emerged as a strong competitor to OpenAI’s o1 series, outpacing it in benchmarks like AIME and MATH. With 32.5 billion parameters and advanced reasoning skills, QwQ-32B challenges the dominance of proprietary models, although it still has quirks.
Meanwhile, OpenAI is responding with a trademark filing for "o1" amidst rising competition from open-source models like QwQ-32B and Nous Research's Hermes 3. Google Labs is reimagining chess with GenChess, blending AI creativity with traditional gameplay. Exciting times ahead!
Alibaba’s QwQ-32B-Preview is turning heads as a heavyweight contender in the reasoning AI arena, aiming for OpenAI’s o1 series. With 32.5 billion parameters and a whopping 32,000-token context window, it’s a mathematical powerhouse, excelling at logic puzzles, advanced math, and planning. Think of it as the valedictorian of LLMs, effortlessly outpacing OpenAI’s o1-preview and o1-mini on benchmarks like AIME and MATH. Alibaba’s innovative approach, including test-time computing for more brilliant inference, shifts the AI game from sheer scaling to architectural finesse. While quirks like language drift and logical missteps keep it from perfection, QwQ-32B’s Apache 2.0 partial release challenges the status quo, signaling that OpenAI doesn’t hold the monopoly on reasoning models anymore.
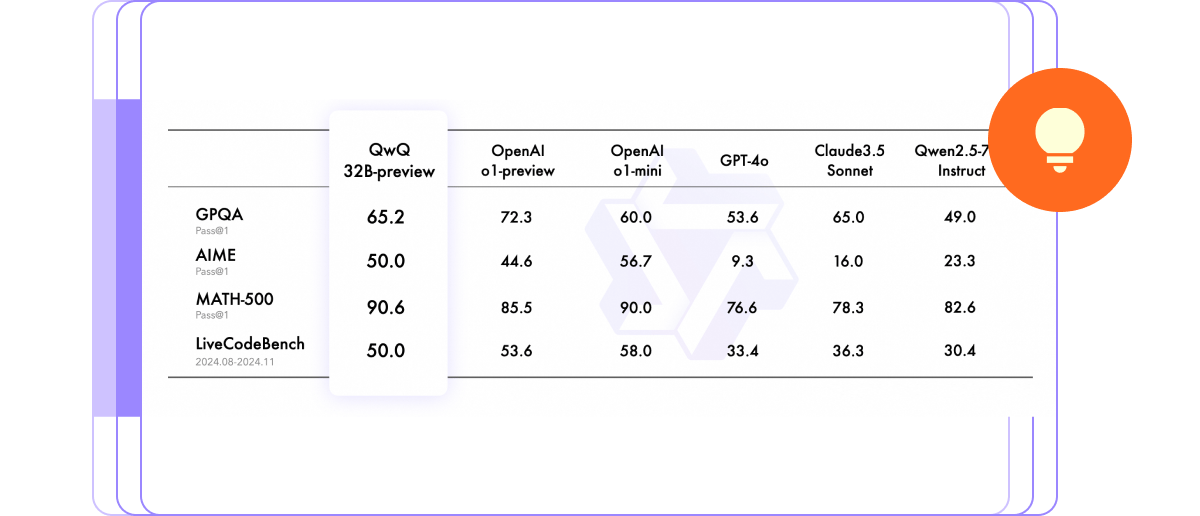
Meanwhile, OpenAI’s moves suggest mounting pressure from rising competitors like QwQ-32B and Nous Research’s Hermes 3. The recent trademark filing for "o1" feels less like routine housekeeping and more like a defensive play. Open-source challengers outperform proprietary systems in critical benchmarks, marking a shift in the AI landscape. As Alibaba and others push for accessibility and innovation, OpenAI’s once unassailable dominance faces cracks in its exclusivity. The race for reasoning supremacy is heating up, and with QwQ-32B leading the charge, the competition is rewriting the rules of the AI game.
Meta's Chief AI Scientist, Yann LeCun, is cautiously optimistic about achieving AGI (Artificial General Intelligence) within the next decade. Known for his skepticism about the current capabilities of LLMs, LeCun has consistently argued that today’s models lack true intelligence, famously comparing them to being “not even as smart as a cat.” However, in a recent podcast, he acknowledged that AGI could emerge in 5–10 years with significant advancements in architecture and systems beyond scaling up LLMs. He emphasized the need for models to learn hierarchically from real-world interactions, dismissing the idea that sheer computational power and data volume alone will bridge the gap to human-level intelligence.

LeCun’s tempered optimism aligns with growing confidence in the AI community. Leaders like OpenAI’s Sam Altman and Anthropic’s Dario Amodei have also suggested AGI could arrive as early as 2026. While LeCun warns of potential technical and unforeseen obstacles, his belief that AGI is within reach signifies extraordinary progress in the field. With bold claims from various AI frontrunners and a clear focus on evolving architectures, the race toward AGI seems more accurate and imminent than ever.

MCP (Model Context Protocol) is here to redefine how developers create AI-driven workflows. Imagine an open standard that turns your AI assistant into a true team player—securely connecting it to your data stack, from Slack to GitHub, without the headaches of custom integrations. By replacing fragmented setups with a universal protocol, MCP empowers developers to focus on building smarter, agentic systems while ensuring secure, scalable data access. And yes, it’s open source because collaboration drives innovation.
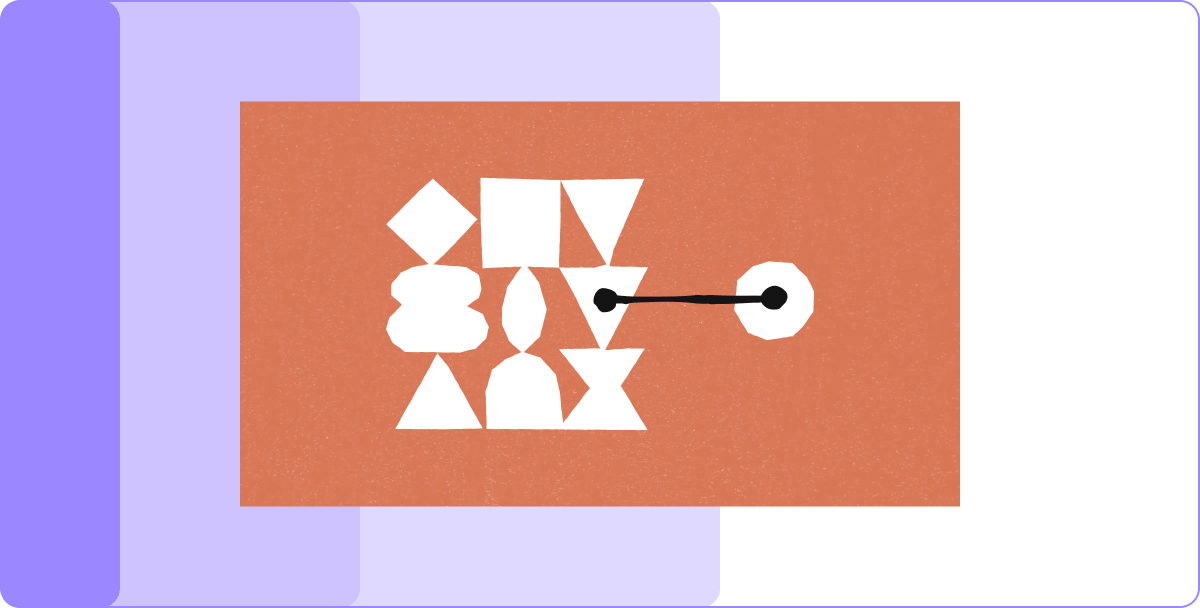
Early adopters like Block and Replit have already showcased how MCP fuels context-aware AI workflows that just work. With pre-built servers, SDKs, and local support in tools like Claude Desktop, developers can quickly build and test secure data connections. Whether you're crafting an AI assistant that understands your dev flow or unlocking insights from siloed data, MCP provides the technical foundation to scale. Ready to let your AI assistant do more heavy lifting? Start with MCP and build the future of open, connected AI workflows.

Google Labs has taken a creative leap with GenChess, an AI-powered spin on the classic game, blending customization and strategy in an undeniably playful way. The experimental platform lets users craft their chess pieces using Gemini Imagen 3, Google’s image generation model. Want sushi-shaped knights or pizza-themed rooks? With a few text prompts and clicks, it’s all possible. Whether you stick with the “classic” look or go “creative” for more whimsical pieces, GenChess promises a fun intersection of AI artistry and gameplay.

Once your masterpiece is ready, you can face off against an AI opponent with its quirky chess set across multiple difficulty levels. For chess purists and AI enthusiasts, the platform offers a glimpse into how design and machine learning can enhance even the most traditional games. And Google isn’t stopping there. Alongside GenChess, the company is deepening its ties to the chess world with initiatives like a Kaggle coding challenge in partnership with FIDE and the upcoming Chess Gem, where Gemini Advanced subscribers can banter with a chess-savvy language model while honing their strategies. Whether you're in it for the sushi pawns or the AI trash talk, it’s clear Google is reimagining the board game for the digital age.

Weekly Research Spotlight 🔍
A Statistical Approach to LLM Evaluation
The paper "A Statistical Approach to LLM Evaluation" offers five key statistical recommendations to improve the accuracy of model performance evaluations. The first suggestion is to use the Central Limit Theorem to measure theoretical averages across all possible questions, not just observed ones. Additionally, it advocates for clustering standard errors when questions are related instead of assuming they are independent to capture the model's performance variability more accurately. It also suggests reducing variance within questions by using techniques like resampling or next-token probabilities.

The paper further emphasizes analyzing paired differences between models to enhance evaluation since questions are often shared across assessments. This allows for more direct comparisons. Finally, the authors recommend using power analysis to determine the appropriate sample size to detect meaningful performance differences between models. By applying these statistical methods, researchers can more accurately assess whether observed performance differences are due to genuine model capabilities or random chance, leading to more reliable and insightful evaluations.

LLM Of The Week
Marco-o1
Marco-o1 is an ambitious attempt to expand upon OpenAI’s o1 model, aiming to address complex real-world challenges that require more than just standard solutions. While OpenAI’s o1 model has made waves with its exceptional reasoning capabilities in structured domains like mathematics and coding, Marco-o1 pushes the envelope by integrating advanced techniques such as Chain-of-Thought (CoT) fine-tuning, Monte Carlo Tree Search (MCTS), and innovative reasoning strategies. These features allow Marco-o1 to explore broader solution spaces and improve its decision-making in real-world tasks without clear-cut answers. By fine-tuning its base model with a mix of CoT datasets and its synthetic data, Marco-o1 aims to tackle better problems with open-ended resolutions, such as those in natural language processing and machine translation.

In its journey to replicate and improve the reasoning abilities of OpenAI’s o1, Marco-o1 has shown promising results, including +6.17% accuracy improvement on the MGSM (English) dataset and +5.60% on the Chinese version. Marco-o1 has also excelled in translating complex slang and colloquial expressions, demonstrating its more profound understanding of language nuance. Using MCTS, Marco-o1 doesn’t simply follow predetermined paths but intelligently explores alternative reasoning directions. The model’s ability to adapt its reasoning granularity, switching between mini-steps and more significant actions, further strengthens its problem-solving power, making it a powerful tool for tackling not just structured problems but also ambiguous, real-world challenges.

Best Prompt of the Week 🎨
Abstract gradient background in monochrome purple tones, smooth flowing shapes, soft lighting, ethereal and dreamy atmosphere, with a gentle blend of light and dark shades of purple


Today's Goal: Try new things 🧪
Acting as an Event Planning Strategist
Prompt: I want you to act as an event planning strategist. You will create a structured daily plan designed to help an individual organize a design challenge and develop a comprehensive sheet of ideas and themes for the competition. You will identify key steps for brainstorming creative concepts, develop strategies for refining themes, select tools to manage submissions and participant engagement and outline additional activities to ensure a successful and inspiring event. My first suggestion request is: "I need help creating a daily activity plan for someone organizing a design challenge and compiling a list of innovative ideas and themes for the competition."

This Week’s Must-Watch Gem 💎

This Week's Must Read Gem 💎
How did you find today's email? |